The cascading risks of bad survey data: Why firms should see quality as a foundation, not a filter The cascading risks of bad survey data: Why firms should see quality as a foundation, not a filter
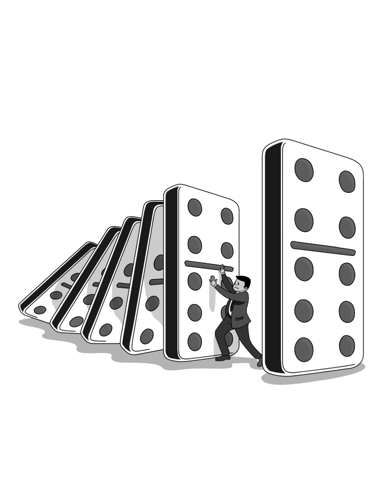
- Reading time
- 1 min
- Words
- Marc Di Gaspero
- Published date
- November 11, 2024
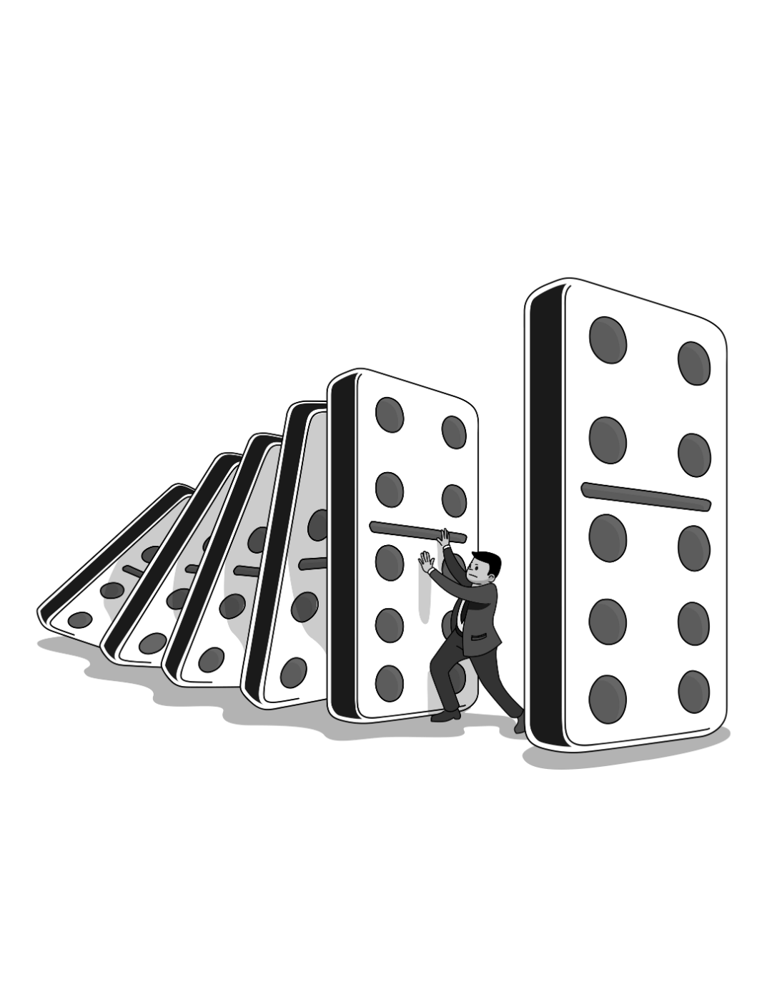
— "Research on research" insights from Marc Di Gaspero, Head of Data Quality at Potloc.
The data quality problem in consulting and private equity.
When flawed data drives decisions in consulting and private equity, the fallout can be severe — investments that don’t deliver, client strategies that don’t land, and thought leadership insights that compromise reputation. IBM estimated that poor data quality cost the U.S. $3.1 trillion in 2016, and that figure is undoubtedly higher today. Yet, in today’s survey ecosystem, ensuring data quality is becoming increasingly challenging — fraud has long plagued the survey industry, with issues like click farms and VPN usage contributing to the challenge. Things have only escalated with the rise of GenAI.
Consulting and PE firms want good data — why else would they invest in primary research? But when the pressure to cut costs and deliver quickly strikes, “quick and dirty” surveys can be alluring, especially as vendors boast about their ability to remove fraudulent or sub-optimal entries. Unfortunately, though, bad data has a way of slipping through, and no amount of cleaning can fully compensate for data that wasn’t sound at the source.
So, what’s the solution? It’s time to shift the focus from filtering out bad data to ensuring data quality from the source. New research on research (RoR) from Potloc shows how a proactive approach to selecting and blending sample sources isn’t just risk mitigation — it’s a hidden competitive edge.
But first, what is data quality?
We define data quality as the collection of reliable and authentic data, achieved when people are honest, attentive, and engaged when taking a survey. This definition is in line with the Global Data Quality (GDQ) Initiative. We also believe that data quality emerges from a combination of three factors:
- The source:
Where are the respondents coming from? As we’ll see, not all supply sources provide equally honest, attentive, and engaged respondents—or involve the same acquisition costs and field times. - The respondent experience:
What kind of experience are you putting survey-takers through? Is it quick and smooth, or repetitive and tedious, without sufficient incentives? The more engaged your respondents are, the more effort they’ll put in, especially on open-ended responses. - Data cleaning:
What measures are in place to monitor responses and filter out inadequate respondents? While this step is essential, it’s equally crucial to recognize that no amount of data cleaning will remove all fraudulent or suboptimal respondents from a sample.
Our study: Measuring how quality, speed, and cost vary across sources.
Recognizing the growing risk of bad data, Marc Di Gaspero, Head of Data Quality at Potloc, set out to conduct a ‘research on research’ study to investigate how different data sources measure up in terms of quality — as well as related factors such as speed, cost, and reach.
The setup:
The study evaluated how five different respondent sources faired in serving up “good” data: honest, attentive, and engaged respondents. To do so, we sampled 6,000 U.S. adults, each of whom had at least one subscription (i.e. streaming service, gym membership, magazine). The sources we compared were:
Potloc designed the study to ensure that all sources were measured on an even playing field. Survey questionnaires and quality checks used were standardized across all sources, leaving only the source itself as the variable impacting data quality. In comparing sources, we used the relative good-to-bad data quality ratio, rather than simply counting the amount of good respondents. This was a more reliable measure as it allowed us to focus on quality differences between sources, not other elements such as survey frequency, overquotas, partials, and ineligibles.
It purposely excludes other final statuses (high-frequency survey takers, overquotas, partials, and ineligibles) to minimize noise.
The final status of a respondent in our study could be one of the following:
Good quality: Completes who successfully pass pre-, in-, and post-survey quality checks.
Active survey taker (15-30): Survey entrants terminated pre-survey because they attempted more 15 to 30 surveys over the last 24 hours as measured by Research Defender's Activity.
Professional survey taker (>30): Survey entrants terminated pre-survey because they attempted more than 30 surveys over the last 24 hours as measured by Research Defender's Activity.
Overquota: Survey entrants terminated in-survey (within the screener) because the quota to which they correspond based on their profile is already full.
Ineligible: Survey entrants terminated in-survey (within the screener) because their profile doesn’t meet the survey’s qualification criteria.
Partial: Survey entrants who drop out of the survey before completing it.
Key findings:
- Quality varies significantly across supply sources.
- Quality sources can require more cost and time investment upfront.
What this means for consultants and PE investors:
- When data collection begins with attentive, honest, and engaged respondents in the first place, data cleaning becomes a refinement process — rather than a rescue operation. This difference can separate insights that make waves from those that just add to the noise. For consulting and PE firms — where speed and accuracy are critical — investing in quality data upfront helps avoid potential data cleaning delays, reputational damage, and compounding costs down the line.
- That said, no source is flawless. Fortunately, market researchers today have developed advanced data cleaning measures that can render almost any sample at least good enough. This is particularly helpful because sourcing decisions aren’t just about quality — reach, speed, and cost vary between sources too. In fact, a thoughtfully curated blend of sources (multi-source sampling) is often necessary to get extra-large or ultra-niche samples, especially when you’re pressed for time and budget.
Building data quality into your process today.
- Demand transparency from your sample providers.
Many suppliers aggregate respondents from external sources without full disclosure. We saw that sample providers (even some “premium” ones) claimed to send proprietary respondents to the survey, but a side investigation by Potloc on 2 panels revealed that only 1-4% of respondents were truly proprietary (i.e. they came from other panels). Multi-source sampling is a reality, but a lack of transparency can compromise the integrity of your data. As a buyer, ask for clear sourcing details. If your supplier can’t provide transparency, it’s a warning sign that your data might not be as reliable as they claim. - Context matters: Tailor decisions to your project’s demands.
Your project’s context dictates the type of data you need. For fast-moving projects with tight deadlines, good data — when triangulated with other sources like expert calls — may be sufficient. But for high-stakes projects that demand unique insights, you need great data. This is where a skilled sample curator comes in as a competitive advantage, carefully blending multiple samples to deliver exactly what your project requires: speed, depth, or a balance of both. - Respondent experience: Another overlooked factor.
Between selecting sample sources and cleaning data lies a crucial but often overlooked aspect — respondent engagement. Engaging respondents from the start helps ensure that the data collected is not only clean but meaningful and actionable, especially in strategic, high-stakes decisions. Both sample providers — and you — have a part to play when it comes to survey design and optimization. By the way: Our next Research on Research study will tackle the impact of respondent experience on data quality.
Strike the balance with Potloc’s sampling experts.
Choosing the right supply source(s) and calibrating for cost, speed, and quality may seem like a chore when you already have other priorities on the go. A comprehensive survey partner can help take the legwork out of respondent sourcing.
Potloc’s platform and experts cater exclusively to the needs of consulting and private equity firms: Just tell us your research goals, and we’ll combine our proprietary sources and vetted partner network sources to deliver the right blend of sources at the right quality, speed, and cost.